Recent research has shown a remarkable 90% accuracy rate in detecting common online threats and DDoS attacks, which is a significant improvement over previous detection methods.
As exclusively reported to Cyber Security News, the new technique operates by keeping a close eye on the constantly shifting internet traffic patterns.
The playbook most frequently used to identify denial-of-service attacks, in which attackers attempt to bring down a website by flooding it with requests, has been modified by scientists.
Denial-of-service threats were discovered in this scenario due to asymmetric behavior in network traffic and Tsallis entropy.
Specifics of the New Technique to Prevent DDoS Attacks
This new technique was created by experts at the Department of Energy’s Pacific Northwest National Laboratory.
Scientist Omer Subasi from PNNL presented the findings on August 2 at the IEEE International Conference on Cyber Security and Resilience, where the article won the award for best research paper presented.
Many systems rely on a raw number called a threshold to identify DDoS attacks. A site’s defenses are activated if the number of people attempting to access it exceeds a certain threshold because it is assumed that an attack is approaching.
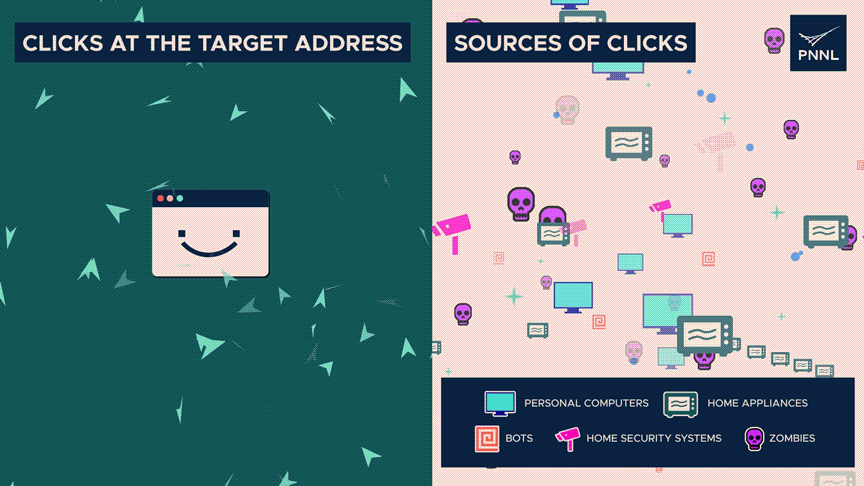
However, relying on a threshold exposes systems to risk.
“A threshold just doesn’t offer much insight or information about what is going on in your system,” said Subasi.
“A simple threshold can easily miss actual attacks, with serious consequences, and the defender may not even be aware of what’s happening.”
A threshold may also produce false alarms, which may have detrimental effects on their own.
The PNNL team fully avoided the idea of thresholds to increase detection accuracy. Instead, the group concentrated on the development of entropy, a metric for system disorder.
Researchers say two measures of entropy move oppositely during a denial-of-service attack.
There are considerably more clicks than typical at the destination address, indicating poor entropy.
However, the origins of those clicks—whether they be from real humans, zombies, or automated systems—are dispersed widely—high entropy. The discrepancy can indicate an attack.
In tests conducted by PNNL, 10 common algorithms properly recognized, on average, 52% of DOS attacks; the top algorithm correctly identified 62% of attacks the PNNL formula accurately recognized 99 percent of them.
To compute entropy, Subasi looked at other methods. Numerous denial-of-service detection systems depend on the Shannon entropy formula.
Instead, Subasi used a mathematical concept called Tsallis entropy for some underlying mathematics.
The Tsallis formula, according to Subasi, is hundreds of times more sensitive than Shannon at identifying false alarms and distinguishing between real flash events like significant internet traffic for the World Cup and an attack.
“The PNNL solution is automated and doesn’t require close oversight by a human to distinguish between legitimate traffic and an attack,” says the report.
The researchers claim that their program is “lightweight” since it just requires a small amount of network or computational resources to do its task.
It has been noted that this differs from solutions based on artificial intelligence and machine learning. These methods avoid thresholds as well, but they also need a lot of training data.
The principal investigator Kevin Barker said, “With so many more devices and systems connected to the internet, there are many more opportunities than before to attack systems maliciously.”
According to reports, the team is currently looking into the potential impact that the deployment of 5G networking and the increasing prevalence of the Internet of Things could have on denial-of-service attacks.
Keep informed about the latest Cyber Security News by following us on GoogleNews, Linkedin, Twitter, and Facebook.